Jeong Hoon Jang
장정훈
Biostatistician
Humanities, Arts, and Social Sciences, Underwood International College
Department of Applied Statistics, College of Commerce and Economics
Yonsei University
Office : Veritas Hall B 434
Tel: +82-32-749-3032
E-mail:jangjh@yonsei.ac.kr
Curriculum Vitae
-
Assistant Professor, Indiana University School of Medicine
-
ORISE Research Fellow, US Food and Drug Administration
Academic Credential
-
PhD in Biostatistics, Emory University
-
BS in Statistics and Mathematics, New York University
Awards/Honors/Memberships
-
Livingston Fellow Award
-
American Statistical Association Student Paper Competition Award
-
Founders Day Award
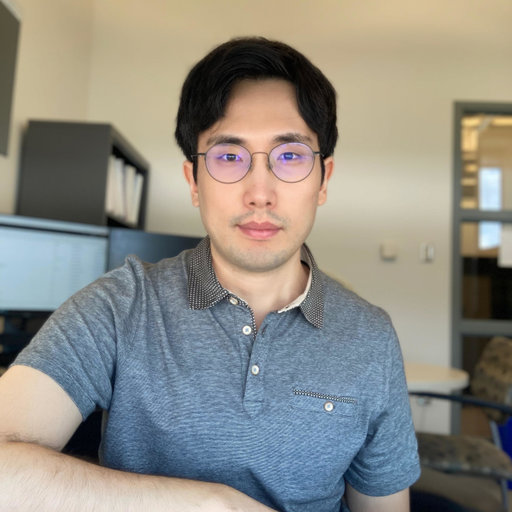
Biostatistics and Health Data Science Research Unit
The Biostatistics and Health Data Science lab develops cutting-edge statistical methods for effectively leveraging information from complex and multi-modal data produced by high-tech medical imaging and wearable device technologies. The overarching clinical goal of the newly developed statistical methods is three-fold:
i) to facilitate discovery, evaluation and validation of novel non-invasive biomarkers
i
i) to improve prediction of disease risk and health condition
iii) to delineate complex pathophysiology of various diseases for their cure and prevention.
The specific data modalities our group studies encompass traditional types that are categorical-, ordinal- and continuous-scaled, as well as those that are highly structured and complex, such as high-dimensional, multi-dimensional, functional (e.g., curve and image) data and their mix.
Current Projects:
1. Develop statistical methods that can effectively analyze color intensity data of patient-sourced fingernail photos to enable non-invasive detection of anemia in infants.
2. Develop statistical methods that leverage glucose level data collected by wearable glucometers to dynamically predict impending adverse events (e.g., hypoglycemia) in diabetic patients.
3. Use renogram image data to non-invasively detect kidney obstruction.
Research Publications (selected)
Jang JH (2021). Principal component analysis of hybrid functional and vector data. Statistics in Medicine, 40(24), 5152-5173.
Jang JH, Manatunga AK, Chang C and Long Q (2021). A multiple imputation approach to bivariate functional data with missing components. Statistics in Medicine, 40(22), 4772-4793.
Chang C, Jang JH, Manatunga AK, Taylor AT and Long Q (2020). A latent Bayesian class model to predict kidney obstruction in the absence of gold standard. Journal of the American Statistical Association, 115(532), 1645-1663.
Jang JH, Peng L and Manatunga AK (2019). Assessing alignment between functional markers and ordinal outcomes based on broad sense agreement. Biometrics, 75(4), 1367-1379.
Jang JH, Manatunga AK, Taylor AT and Long Q (2018). Overall indices for assessing agreement among multiple raters. Statistics in Medicine, 37(28), 4200-4215.